
Well, the best answer we have found so far is the National Sample Survey of Registered Nurses.
"Registered nurses sampled answer questions on their education and training in nursing, professional nursing certifications, education and workforce participation prior to becoming a registered nurse, current and recent workforce participation, income, demographic characteristics, and States in which they hold current licenses."All sorts of great socio-economic data here. Very fantastic.
There are two public-use files available here from various years, 2000 being the most recent. We accessed the county-level 2000 file. Downloaded the zipped data to find an non-delimited ascii file, in addition to codebooks and a couple SAS scripts. We do not have SAS loaded in the GIS lab (to this point SPSS has been sufficient), so I made my way to another lab with SAS loaded and spent the better part of a morning building the SAS table with the provided scripts and then exported the data into a delimited format usable by ArcGIS. Then, of course, I ran it through my replacer program, one of the first programs I ever wrote. This little program uses regular expressions to remove all characters from the first line of a coma-delimited file except for alphanumeric characters (easily does this using the /w expression) so that ArcGIS can import the data. Then I imported the table into an Access database.
If anyone ever needs to do similar operations, here is the Access database I generated.
The website specifies the public-use county files identify the county and metropolitan areas that the nurses currently employed. However, as you can see in the database I generated, there is also a field for the city of current employment. I created a relationship in the Access database between the nursing sample data and the TIGER designated places table and got 7,246 matches! This means the 35,579 respondents in the public-use file can now be joined in ArcMap to particular 7,246 places!
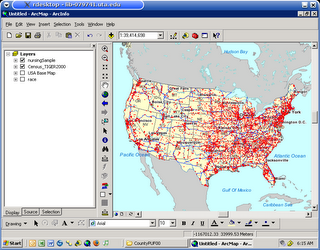
Now, concerning the race data that started this whole process, this datasets falls a tad bit short. I only found one field [RACE_GP] that contains the following coded values:
1 = "WHITE"
2 = "OTHER"
-9 = "UNKNOWN/REFUSED"
-6 = "DECEASED"
The professor is in the process of contacting the HRSA to see if they are willing to provide more data.
Either way, this dataset is a keeper and I am very happy to have come across it.